Test AI on YOUR Website in 60 Seconds
See how our AI instantly analyzes your website and creates a personalized chatbot - without registration. Just enter your URL and watch it work!
Introduction to AI and Data Privacy
Data privacy refers to the protection of personal information from unauthorized access, misuse, or exposure. AI systems often require extensive datasets to function effectively, leading to potential risks such as data breaches, identity theft, and algorithmic biases. As AI continues to advance, it is essential to balance innovation with ethical and legal considerations to ensure user data remains secure.
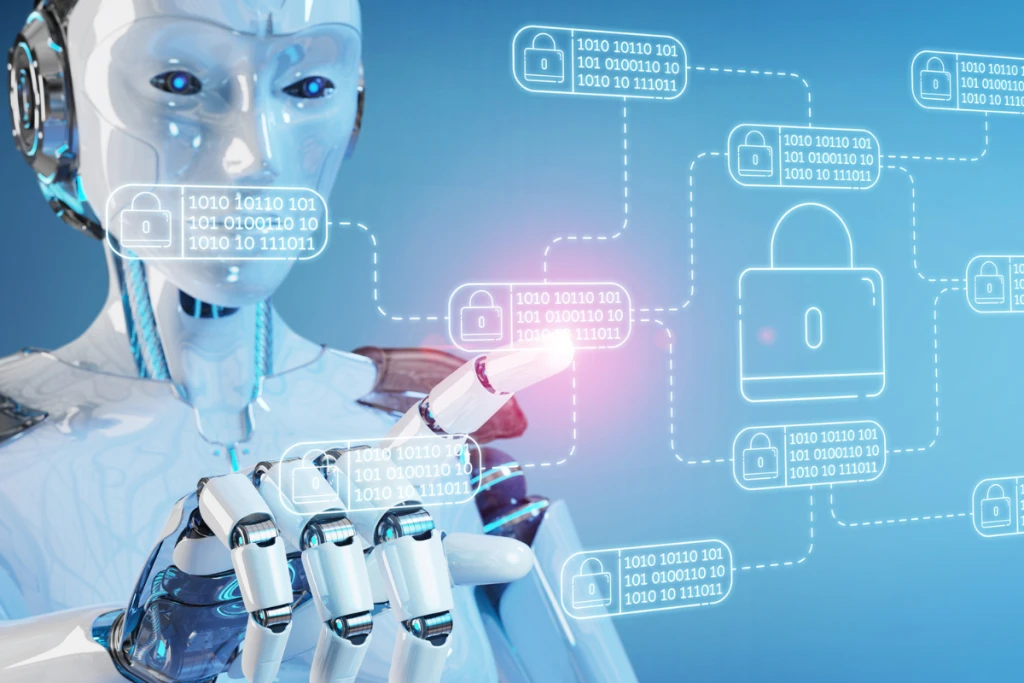
Privacy Challenges in the Age of AI
Massive Data Collection
Many AI applications, such as recommendation systems, facial recognition technology, and voice assistants, require large amounts of data to improve their accuracy and performance. This leads to continuous data collection from users, often without their explicit knowledge or consent. Social media platforms, for example, track user interactions to refine their algorithms, but this practice can blur the line between personalized experiences and invasive surveillance.
Lack of Transparency
One of the biggest concerns with AI is its "black box" nature. Many AI-driven decisions are not easily explainable, making it difficult for users to understand how their data is being used. If an AI model denies a person a loan or a job opportunity based on its analysis, the affected individual may have no way of understanding or challenging the decision. This lack of transparency can undermine trust in AI systems and raise ethical concerns.
Bias and Discrimination
AI systems are trained using historical data, which may contain inherent biases. If not carefully managed, AI models can perpetuate or even amplify discrimination. For example, biased facial recognition systems have been found to misidentify individuals from certain demographic groups at higher rates. This not only raises ethical concerns but also legal risks for businesses relying on AI-based decision-making.
Enhanced Surveillance
AI-driven surveillance tools, such as facial recognition and behavior tracking, are becoming increasingly prevalent. While these technologies can enhance security, they also pose serious threats to privacy. Governments and corporations can use AI to monitor individuals without their consent, raising concerns about mass surveillance and potential misuse of personal data.
Best Practices for Protecting Personal Data in AI Applications
Data Minimization
Organizations should collect only the data necessary for their AI applications. Reducing the amount of stored personal information minimizes the risk of data exposure in the event of a breach.
Data Masking and Pseudonymization
Techniques such as data masking (replacing sensitive data with fictitious values) and pseudonymization (removing direct identifiers from datasets) can enhance privacy while still allowing AI models to function effectively.
Informed Consent and User Awareness
Users should have clear and accessible information about how their data is collected, used, and stored. Implementing opt-in policies rather than automatic data collection ensures greater transparency and user control.
Regular Security Audits
AI systems should undergo frequent security audits to identify vulnerabilities and potential privacy risks. This includes testing for data leaks, unauthorized access, and bias detection.
Robust Encryption Protocols
Encrypting stored and transmitted data adds an extra layer of security, making it more difficult for unauthorized parties to access sensitive information.
Regulatory Frameworks and Compliance
General Data Protection Regulation (GDPR)
Enforced by the European Union, GDPR sets strict guidelines on data collection, storage, and user consent. Companies must provide transparency regarding data usage and allow individuals to request data deletion.
California Consumer Privacy Act (CCPA)
This U.S. regulation grants California residents greater control over their personal data, requiring companies to disclose data collection practices and provide opt-out options.
AI-Specific Ethical Guidelines
Several organizations, including the OECD and UNESCO, have introduced ethical AI guidelines emphasizing transparency, fairness, and accountability in AI development and deployment.
The Role of Organizations in Ensuring Data Privacy
Developing Ethical AI Frameworks: Establishing internal guidelines for AI development that prioritize user privacy and ethical considerations.
Training Employees on Data Protection: Educating staff on best practices for data security and compliance with privacy regulations.
Implementing Privacy by Design: Integrating data protection measures at the development stage of AI projects rather than as an afterthought.
Engaging in Transparent Communication: Providing clear explanations to users about how their data is used and ensuring they have control over their information.
Future Outlook: Balancing Innovation and Privacy
Federated Learning: A decentralized approach to AI training that allows models to learn from data without transferring it to a central server, enhancing privacy.
AI Regulation and Ethical AI Development: Governments worldwide are expected to introduce stricter AI regulations to prevent misuse and ensure data protection.
Greater User Control Over Data: Emerging technologies may offer individuals more control over their personal data, such as self-sovereign identity systems using blockchain.